The Foundation of Successful AI Integration
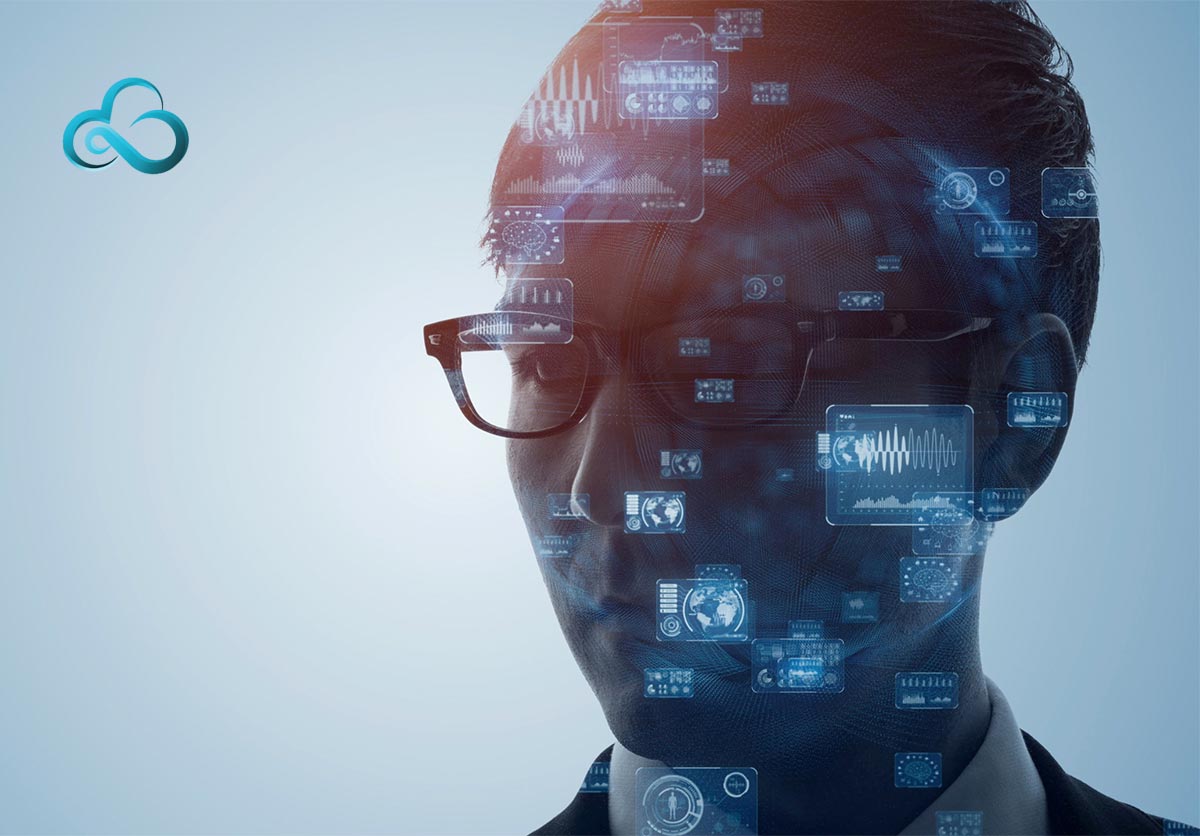
We’ve previously covered how businesses can be transformed by AI, but only if there’s good data to work with. Before you can use AI, it’s super important to evaluate your data sources carefully. It’s all about having enough data, following privacy rules, and improving data quality if needed. This blog will cover the significance of data for AI, how to evaluate its availability and quality, and optimising your data for AI applications.
Understanding the Importance of Data for AI
AI algorithms love data. The more data they get, the better they are at making accurate predictions and decisions. Data is like the building blocks of AI. It helps it learn patterns, spot trends, and give valuable insights. Without good data, even the fanciest AI models won’t give you any useful results.
Data can come from different sources in your business, like customer interactions, sales, operations, and outside datasets. For AI to work its magic, you need loads of accurate, relevant data that plays by the rules.
Evaluating Data Availability
First, check if your data is available. Check if the data you have can support your AI initiatives.
- Identify Data Sources: Start by listing all the data sources within your organisation. These could include CRM systems, customer support logs, sales records, marketing databases, and more. Consider both structured data (e.g., databases, spreadsheets) and unstructured data (e.g., emails, social media posts).
- Assess Data Volume: Determine whether you have enough data to train your AI models effectively. AI algorithms need loads of data to learn accurately. If you don’t have enough data, think about ways to increase it or find more sources.
- Evaluate Data Coverage: Ensure that your data covers all relevant aspects of the business problem you are trying to solve. For example, if you are implementing AI for customer support, your data should include customer queries, response times, resolution outcomes, and customer satisfaction scores.
Assessing Data Quality
AI can only reach its full potential with high-quality data. To have good data quality, your data has to be accurate, complete, consistent, and reliable. Here’s how to check and boost your data quality.
- Accuracy: Verify that your data is accurate and free from errors. Inaccurate data can lead to incorrect AI predictions and decisions. Regularly validate your data against reliable sources to ensure its accuracy.
- Completeness: Check that your data is complete and does not have missing values. If there’s missing data, AI models might not work well. Figure out ways to fill in missing values or use data imputation techniques.
- Consistency: Ensure that your data is consistent across different sources and formats. Inconsistent data can create challenges for integration and analysis. Standardise formats and establish data governance policies to maintain consistency.
- Timeliness: Assess the timeliness of your data. Using outdated data can give you wrong AI insights. Try to use real-time data collection and processing so your AI models work with the latest info.
Data Privacy and Compliance
Data privacy and compliance are super important when checking your data for AI. You need to make sure your data practices are legal and respect user privacy.
- Data Consent: Ensure that you have obtained the consents from individuals for data collection and processing. Clearly communicate how their data will be used and provide options to opt-out if desired.
- Anonymisation and Encryption: Protect sensitive data through anonymisation and encryption techniques. Anonymisation removes personally identifiable information (PII) from your data, while encryption secures data in transit and at rest.
- Data Access Controls: Implement strict access controls to ensure that only authorised personnel can access sensitive data. Regularly review and update access permissions to maintain data security.
- Compliance Audits: Conduct regular compliance audits to ensure that your data practices adhere to relevant regulations. Stay updated with changes in data protection laws and adjust your policies accordingly.
- Investing in Data Cleaning and Enrichment
If your data quality isn’t great, you really need to invest in cleaning and enriching it for better AI performance. Data cleaning means finding and fixing errors, and data enrichment means improving your data by adding useful information.
- Data Cleaning: Implement automated data cleaning tools to identify and correct inaccuracies, remove duplicates, and standardise data formats. Regularly monitor and maintain data quality to prevent issues from arising.
- Data Enrichment: Enhance your data by adding external data sources or additional attributes that provide more context. For example, enriching customer data with demographic information or social media activity can improve AI-driven personalisation.
- Data Integration: Integrate data from different sources to create a unified dataset. Use data integration tools and platforms to streamline this process and ensure that your AI models have access to comprehensive and cohesive data.
Building a Data Strategy
To effectively manage data availability and quality, it’s essential to develop a comprehensive data strategy. This strategy should outline how you will collect, store, manage, and use data to support your AI initiatives.
- Data Governance: Establish a data governance framework that defines roles, responsibilities, and processes for data management. This framework should include policies for data quality, security, privacy, and compliance.
- Data Architecture: Design a data architecture that supports efficient data collection, storage, and processing. Consider using cloud-based data platforms that offer scalability and flexibility for AI workloads.
- Data Management Tools: Invest in advanced data management tools that automate data cleaning, enrichment, integration, and monitoring. These tools can significantly reduce the time and effort required to maintain high-quality data.
- Training and Awareness: Educate your employees on the importance of data quality and the role they play in maintaining it. Provide training on data management best practices and encourage a data-driven culture within your organisation.
Leveraging AI for Data Management
Interestingly, AI itself can improve data availability and quality. AI-powered data management tools can automate data cleaning, detect anomalies, and predict missing values, making the process more efficient and accurate.
- Automated Data Cleaning: Use AI algorithms to identify and correct data errors. Machine learning models can learn from historical data corrections and apply similar fixes to new data entries.
- Anomaly Detection: Implement AI-based anomaly detection to identify unusual patterns in your data that may show errors or fraud. This helps in maintaining data integrity and reliability.
- Predictive Data Imputation: Use AI to predict and fill in missing values based on patterns observed in the existing data. This ensures that your datasets are complete and ready for AI training.
Make sure you have reliable data before you use AI. Look at your data sources, make sure they’re reliable, follow privacy regulations, and invest in cleaning and enhancing the data. That way, you’ll have a strong data foundation for your AI projects. Don’t forget, good data makes AI awesome and helps businesses succeed. Stay tuned for the next blog in this series, where we’ll explore how to tell if your business is ready for AI and set a firm foundation for successful implementation. Curious already to know where your business sits? Let’s have a chat.